Looking around these days, it looks like generative artificial intelligence is everywhere, even if it is hard to grasp its concept.
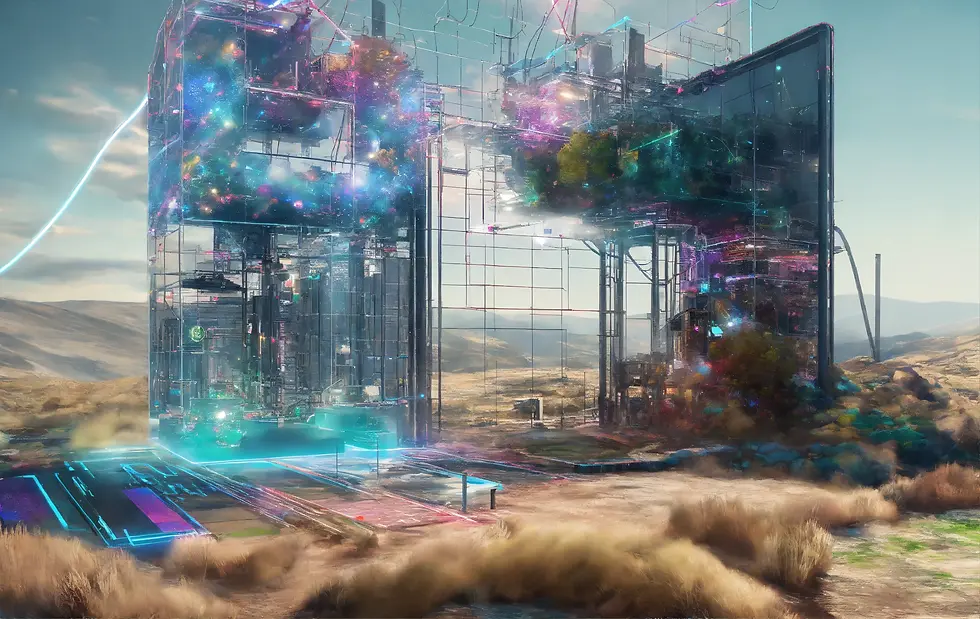
Actually, what is “Generative AI”?
Since the boom of generative AI over the past few years, we would typically talk about AI in general. AI was, and still is, and definitely will be, the most spoken topic in tech, more typically mentioning the machine-learning models that can learn to make a prediction based on data. These models - a term used to describe the type of AI in the topic of discussion - are trained using millions of examples. These predictions could be someone borrowing money from a bank who is likely to default on a loan or even a certain X-ray that could show signs of a tumor.
The main idea of artificial intelligence in a particular field (in the medical field, or even the financial field can benefit from AI) is to teach the machine and let it make the decisions by itself - Machine learning - and give a specific output - Generative AI - whether or not a human will oversee its final predictions.
An increase in complexity
Machine learning can go back more than 50 years. An example of this tech is a model known as the Markov chain. Named by the mathematician Andrey Markov, it introduces a method of statistics on the behavior of random processes. With these processes, AI models have been used for next-word prediction tasks, like the autocomplete function in an email program.
In the past few years, researchers have tended to focus on finding a machine-learning algorithm that makes the best use of a specific dataset. But that focus has shifted, and many researchers are now using larger datasets with hundreds of millions or even billions of data points to train models that can achieve impressive results.
Similar systems from ChatGPT work almost in the same way as the Markov model. The difference is that ChatGPT is far larger and more complex, with billions of parameters trained in one of the biggest data sources, the Internet.
The model of ChatGPT learns the patterns of the block of texts and uses this knowledge to propose the next upcoming text. Then, this would take the sequence of text with its connections in meaning and cut the text into statistical chunks that have some predictability.
Machine learning can go back more than 50 years. Before it was tought on small database, but now it is tought on hundreds of million of data.
A range of applications
For the principal approach, the main idea of all of these models is that they convert the inputs (user information sent to the model or the database from the model already pre-added into the model) into tokens, which are numerical representations of chunks of data. In theory, as long as this data can be converted into this token standard, the AI could generate new data that would look similar.
This model opens up an array of applications for Generative AI. For example, feeding the model with synthetic data ‘tokens’ by teaching the computer vision model to recognize objects using cameras or rays.
According to the researcher Devavrat Shah from MIT, “The highest value they have, in my mind, is to become this terrific interface to machines that are human friendly. Previously, humans had to talk to machines in the language of machines to make things happen. Now, this interface has figured out how to talk to both humans and machines,”.
Raising red or green flags?
Generative AI has incredible potential to learn from training data and create content that reflects diversity and inclusivity. By adding different ‘tokens’ in the training process, bias can be minimized, and the resulting generated content can become more truthful and representative.
Actually, the ability of generative AI to mimic human creators can actually be a positive attribute. It opens up new possibilities for collaboration between humans and AI, enabling creators to leverage AI technologies to amplify their own unique styles and ideas. This collaboration can boost innovation and amplify the creative process by showing new inspirations.
Additionally, generative AI can contribute to addressing the issue of copyright infringement. By providing clear attribution and ownership information, AI-generated content can help prevent plagiarism and ensure proper credit is given to the human creators whose work it may resemble. This opens up opportunities for respectful collaboration, knowledge sharing, and the development of new, transformative works.
Ultimately, by actively addressing potential challenges and working towards the responsible use of generative AI, we can make use of its positive capabilities to foster creativity and boost your tasks, working alongside you, not for you.
“There are differences in how these models work and how we think the human brain works, but I think there are also similarities. We have the ability to think and dream in our heads, to come up with interesting ideas or plans, and I think generative AI is one of the tools that will empower agents to do that, as well,” Phillip Isola, MIT teacher and researcher, says.
Do you think that we should invest ourselves in this era of AI knowledge or continue to work in a slow and steady pace?
Comments